A Guide To Machine Learning: Everything You Need To Know
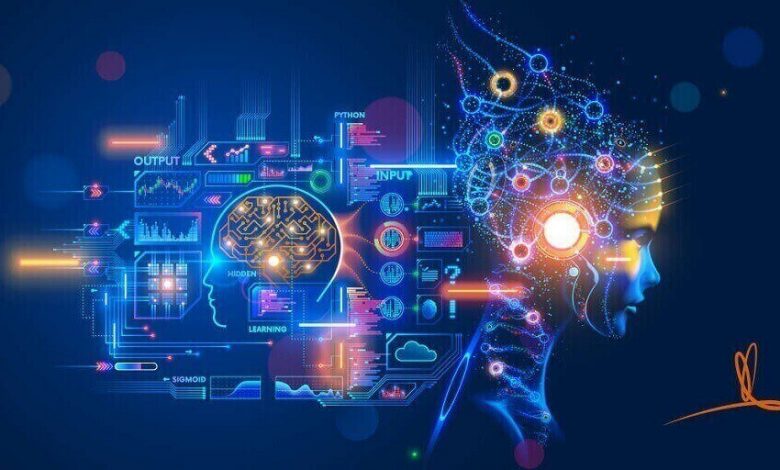
Several tech companies are investing in machine learning to build AI platforms, while startups are focused on niche domain solutions. AI strategies such as deep learning are gaining considerable momentum in the industry as a result of this exponential rise. This blog is intended for new learners who are interested in knowing the basics of machine learning technology. This session discusses the overall summary of ML concepts which covers the concepts like what is ML, what is the purpose of ML, applications of ML, how machines do learn, what are various types of ML, etc. Now go through concepts in detail.
What is Machine Learning?
Machine learning, as the name implies, is a method by which machines learn from and interpret data in order to predict outcomes. It may be classified as supervised, unsupervised, semi-supervised, or unsupervised. Machine learning is a stepping stone to artificial intelligence; it learns from algorithms built on databases and uses them to extract answers and correlations.
What is the Purpose of Machine Learning?
Automation and digital transformation are inextricably linked, and machine learning is at the core of both. Google released the graph-based machine learning platform in 2016. It connected clusters of data based on their similarity using the semi-supervised learning approach. Machine learning technology aids businesses in identifying industry dynamics, risks, consumer demands, and corporate insights. Business intelligence and automation are the norms today, and machine learning is the basis for achieving objectives and increasing the company’s productivity.
Machine Learning Applications
Here’s a short summary of what machine learning can do before we get started:
- Healthcare: Forecasting patient diagnoses for analysis by specialists.
- Social Network: Forecasting those match preferences for improved compatibility on a dating website.
- Finance: Forecasting a credit card’s unauthorized conduct.
- E-commerce: Customer turnover forecasting.
- Biology: Identifying patterns of gene mutations that could indicate cancer.
What approaches do machines use to learn?
Simply put, machines “learn” by analyzing the patterns in similar data. Consider data to be the information you collect from the outside world. A system becomes “smarter” as more data is provided into it. However, not all information is compiled equally. This knowledge has the potential to take you in the right or wrong way. The higher the quality of the data/information collected, the lower the level of uncertainty, and vice versa. As a matter of fact, it’s important to consider the kind of data you’re providing your machine to learn from.
Nevertheless, the machine will make predictions after a reasonable amount of data is provided. Machines will forecast the future as long as it isn’t too dissimilar from the past.
Machines “learn” by analyzing historical data in order to determine what is most likely to occur. If the old data resembles the new data, the observations you might tell about the old data are likely to apply to the new data as well. It’s as if you’re looking backward in order to look ahead.
Machine Learning Types
Machine learning is classified into three classes:
- Supervised learning: Labeled data is used to instruct the machine. Humans are typically responsible for labeling the data.
- Unsupervised learning: Unlabeled data were used to train the machine. In other words, there is no “right” solution for the machine to learn; instead, the machine must try to identify correlations in the data to come up with a solution.
- Reinforcement learning: A reward-based mechanism aids the machine’s learning.
Supervised Machine Learning
Since training a machine to learn with labeled data is easier than training it to learn with unlabeled data, supervised learning is the most prevalent and well-studied form of learning. Supervised learning can solve two kinds of problems: regression and classification, depending on what you want to predict.
Regression:
Regression is used because you choose to estimate “continuous values”, such as the expense of a house or the temperature outside in degrees. Since the value can be any number with no constraints, this form of issue does not have a particular value restriction.
Classification:
You will be using classification if you were to forecast “discrete values,” such as categorizing anything. The response to a question like “Will he make this purchase?” will fall into one of two categories: yes or no. A binary classification issue is another name for this.
Unsupervised Machine Learning
Unsupervised ML aims to find trends in data and group them when there is no labeled data for machines to learn from. This type of learning refers to machines that are attempting to learn “on their own,” without the assistance of a human. This type of learning can combine data by clustering or association depending on whether you choose to group them.
Clustering Problem:
Unprofessional learning can combine data by clustering or association depending on whether you choose to group them. If a particular cluster or group exists, the algorithm can categorize them in a specific way. Attempting to group consumers based on previous purchasing activity is an example of this.
Association Problem:
Unsupervised learning attempts to resolve this issue by attempting to comprehend the laws and meanings underlying various categories. A typical example of a connection problem is determining a connection between consumer transactions. Markets will want to know what items were bought together and may use this knowledge to arrange product placement for better access.
Reinforcement Machine Learning
A reward/penalty system is needed for this form of machine learning. When the computer learns correctly, it is rewarded; when it learns incorrectly, it is fined.
Artificial Intelligence is a branch of Reinforcement ML. Since there are so many different explanations from the data, this method of learning is an iterative process. It continuously learns.
Hyperautomation’s Role in Machine Learning
Hyper Automation, as described by Gartner, is the world’s newest technology trend. It allows businesses to automate more of their procedures and obtain intelligent, real-time feedback from the data they gather. Some of the main developments behind hyperautomation’s growth include machine learning, artificial intelligence, and robotic process automation (RPA). It aids AI’s ability to augment human behavior. When equipped, ML algorithms can automate a variety of tasks. Machines and applications will be able to automatically upgrade and adapt to changes based on market needs due to ML models and AI.
How are industries using machine learning?
As per Industry Research, between 2020 and 2024, the global machine learning market is expected to expand by USD11.16 billion, with a CAGR of 39%.
This information is sufficient to demonstrate the development and adoption of ML around the world. How ML is used in various industries, let’s have a look.
- It has aided a number of future advances in the healthcare field. ML was used in the vaccine development process during the Covid-19 pandemic, with various models defining protein structures and correlating them to their potential effect on patients. As compared to traditional approaches, ML in drug exploration saves time and money. It has a lot of potential in the healthcare and pharmaceutical industries, from automating routine processes to keeping Electronic Health Records to diagnosing various diseases.
- With the exponential growth of eCommerce and online shopping, companies are looking for new technology to streamline their operations and better serve their customers’ needs. Businesses, particularly eCommerce and retail, are becoming more customer-centric, and ML plays a key role in this. It is helping in tracking customer needs and trends, targeted ads and digital marketing, personalization of services, supply chain management, predicting customer behavior, etc.
Banking and finance, automobiles, manufacturing, cybersecurity, press, and many other industries are using machine learning. You can get this online Machine Learning Training which provides practical in-depth knowledge on the topics which you have gone through in this session.
Conclusion
We hope you have understood and have gone through this information provided which assists you as a prerequisite knowledge while learning this technology.